What is text mining and how does it drive value from unstructured text?
We know that every customer response, comment, review, and opinion contains valuable insights with the potential to significantly impact a company's success. However, due to the sheer volume and unstructured nature of this data, it can be overwhelming to extract meaningful information.
This is where text mining emerges as a powerful tool, providing a systematic approach to uncovering the valuable information hidden within textual feedback.
This article dives into text mining, a process that leverages algorithms to extract actionable insights from texts such as customer feedback, social media posts, reviews, and documents. We will discover the methods used, the benefits for businesses, and real-world examples of text mining in action.
TL;DR
- Text mining extracts insights from unstructured text data like customer feedback through methods like natural language processing.
- It involves steps such as data preparation, cleaning, and extracting information using techniques like sentiment analysis.
- Businesses utilize text mining to enhance customer experience, identify trends, and make data-driven decisions.
- Automation and proper tool selection optimize text mining operations, saving time and improving efficiency.
- Text mining empowers businesses to gain a competitive edge by understanding market dynamics and customer preferences.
- With Zonka Feedback, businesses can capture customer feedback to identify relevant data and get information retrieval for data-driven decision-making. You can sign up for a free 14-day trial.
Measure Customer Feedback & User Insights
With powerful surveys, understand what users need and learn ways to delight your customers.
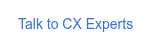
Demystifying Text Mining: The Basics
Text mining acts as a bridge between human language and the comprehension of machines by automating the transformation of unstructured text into structured data for efficient analysis.
At its core, text mining is the process of extracting meaning and knowledge from unstructured textual data. It's like having a superpowered reading machine that analyzes vast amounts of text, identifying patterns, trends, and hidden gems.
Essentially, it involves organizing unstructured information and extracting valuable knowledge through techniques such as NLP (natural language processing) and Machine Learning. NLP enables understanding of human language and Machine Learning offers predictions & trends by learning from the text data.
This process is crucial in making sense of natural language and turning it into actionable insights from previously disorganized data.
Understanding Unstructured Data
Unstructured data is characterized by its lack of organization or predefined format. It can take many forms, including news stories, job listings, movie reviews and social media posts. Despite being difficult to manage initially, unstructured text data holds great value for businesses as it allows them to uncover patterns in customer behavior and gain valuable insights.
The challenge lies in converting this unstructured text into a structured format that enables effective analysis. This transformation process is essential as it ultimately unlocks the potential of unstructured data for organizations.
In customer feedback, unstructured data could be in different formats.
- Open-ended questions: These invite respondents to answer in their own words, providing valuable insights beyond fixed options. Examples include:
- "What did you dislike most about the product?"
- "What suggestions do you have for improvement?"
- "Please describe your overall experience with our service."
- Comments and feedback boxes: While these may often focus on specific questions, they leave room for additional thoughts and feedback not captured by other response options.
- Text responses to rating scales: While the rating itself might be structured (e.g., 1-5 raing scale, 5-stars, Likert Scale), the accompanying text can offer valuable context and reasoning behind the rating.
- Images and multimedia: Some surveys might collect pictures, audio recordings, or videos, requiring specific tools for analysis beyond traditional text analysis.
- Social media conversations: Surveys embedded in social media platforms might capture unstructured responses and comments alongside structured data like demographics.
The Role of NLP (Natural Language Processing) in Text Mining
Natural Language Processing (NLP) can be seen as the savior for text mining, taking on the role of a knight in shining armor. With NLP, computers are able to understand and respond to both written and spoken human language.
Through its ability to interpret data from natural language, NLP is instrumental in transforming unstructured text information into organized formats. This enables efficient sorting, extracting meaningful insights and creating summaries out of scattered pieces of data.
In fact, using machine learning helps natural language understanding and makes it easier to idenitify relevant data and share actionable insights to take action.
Benefits of Text Mining: Powering Businesses
Now that the basics of text mining have been understood, it is important to delve into its potential benefits for businesses. Through text mining, companies can utilize customer feedback, social media posts, and call center transcripts to gain actionable insights.
By identifying patterns and trends within this data, informed decisions can be made in order to optimize performance and strengthen risk management strategies on various platforms including social media.
Text mining customer feedback unlocks a goldmine of hidden knowledge, empowering businesses to make data-driven decisions and ultimately improve the customer experience. Here are some key advantages and benefits:
1. Deeper Customer Understanding
Text mining allows businesses to uncover hidden emotions, nuances, pain points, and issues behind customer feedback. It gives them valuable insights and helps them understand what they are actually feeling.
- Beyond surface-level responses: Go beyond simple ratings or "yes/no" answers to uncover true motivations, preferences, and pain points.
- Identify hidden trends and patterns: Discover common themes and issues raised across various feedback channels, providing a holistic view of customer sentiment.
- Segment and personalize experiences: Analyze feedback based on demographics, purchase history, or other factors to tailor products, services, and marketing to specific customer segments.
2. Boosting Customer Experience, Satisfaction, and Loyalty
The advantages of text mining extend to the potential for improving customer experience. As companies get a deeper understanding of their customers, they can clearly identify their preferences and issues. This valuable information can then be utilized to enhance products and services, ultimately leading to improved experience, satisfaction, and loyalty.
- Proactive and personalized customer service: Analyze feedback to anticipate customer needs and provide relevant support before issues arise.
- Improved complaint resolution: Understand the root cause of customer dissatisfaction and address it effectively, preventing repeat issues and building trust.
- Measure and track customer sentiment: Monitor overall customer satisfaction over time and identify areas for improvement.
3. Improved Products and Services
Customer feedback often contains valuable suggestions for product improvements or new features. Text mining allows businesses to systematically analyze this feedback and prioritize product enhancements based on customer demand, leading to more competitive and customer-centric offerings.
- Identify product flaws and improvement opportunities: Understand what customers love and hate about your products, informing future iterations and development.
- Proactive issue detection and resolution: Uncover emerging problems before they escalate, addressing them swiftly and preventing customer churn.
- Prioritize development efforts: Base product roadmap decisions on actual customer feedback and needs, ensuring resources are allocated effectively.
4. Enhanced Marketing and Sales
Businesses that effectively leverage text mining from customer feedback can gain a competitive edge by being more responsive to customer needs and market trends. They can identify opportunities and enable businesses to strategize data-driven marketing and sales campaigns.
- Targeted campaigns and personalization: Analyze feedback to understand customer preferences and tailor marketing messages and offers for higher engagement and conversion rates.
- Discover effective messaging and language: Learn what resonates with customers and use that knowledge to craft compelling marketing materials and sales pitches.
- Identify key influencers and brand advocates: Find customers who consistently rave about your products and leverage their testimonials for social proof and word-of-mouth marketing.
The Text Mining Techniques & Process: From Raw Data to Insights
As we recognize the significance of text mining, it is necessary to explore how unprocessed data transforms into important understandings. The process of text mining comprises various stages such as preparing the data, cleaning it, and extracting information from it.
Each stage plays a crucial role in guaranteeing that the data for analysis is precise and reliable.
To effectively transform raw feedback data into valuable insights, a step-by-step process is essential. The following steps outline how text mining can be used to analyze customer feedback and derive actionable conclusions:
#Step 1. Data Gathering: The first step in text mining is to collect customer feedback from various sources such as surveys, reviews, social media comments, and support tickets. This allows businesses to gather a diverse range of opinions and perspectives.
#Step 2. Data Cleaning & Prepping: Once the data is gathered, it needs to be cleaned and prepped for analysis. This involves removing irrelevant information and standardizing formats. By doing so, businesses can ensure that the data is accurate and can be effectively analyzed.
#Step 3. Text Processing: After cleaning the data, the next step is to break down the text into manageable chunks such as words and phrases. This includes tokenization, stemming/lemmatization, and part-of-speech taging. Additionally, tagging these chunks with their grammatical roles, such as nouns and verbs, can provide further context and insights.
#Step 4. Extracting Information: The next step after preparing and cleaning the training data is to extract information using text mining methods. These techniques, which include natural language processing (NLP), information extraction, and information retrieval, are used to identify and extract important entities and concepts from textual data.
These enable computers to understand human language better and uncover insights such as sentiment analysis, summarization, and aspect mining. This process makes use of NLP tools that facilitate comprehension of natural language for tasks like retrieving relevant information from a given text.
#Step 5. Feature Extraction: Identifying key elements within the text is crucial for deriving meaningful insights. This includes extracting information such as word frequency, sentiment (positive, negative, neutral), and specific topics discussed. By analyzing these features, businesses can understand the overall sentiment and prevalent themes within the feedback data.
#Step 5. Analysis & Modeling: To uncover trends and patterns within the feedback data, businesses can utilize various text mining tools and techniques. Sentiment analysis can help identify the overall sentiment of the feedback, topic modeling can group similar comments together, opinion mining can identify opinions behind the text, intent analysis can tell the basic intention and entity recognition can identify specific entities mentioned.
These analyses provide a deeper understanding of the feedback data and highlight important patterns.
#Step 6. Insights & Action: The final step in the text mining process is to translate the findings into actionable steps. These insights can be used to drive product improvements, create targeted marketing campaigns, and enhance personalized customer service interactions. By taking action based on the insights gained from text mining, businesses can improve customer satisfaction and mitigate potential risks.
By following these steps, businesses can effectively analyze and derive valuable insights from raw feedback data. Text mining enables businesses to unlock the potential within unstructured textual information and make data-driven decisions to improve customer satisfaction and support risk management.
Text Mining vs. Its Cousins: Clarifying the Confusion
While text mining, text analysis, and data mining may seem similar in name, each has its own specific focus and practical uses.
Data mining is primarily concerned with examining organized or structured data.
On the other hand, text mining deals specifically with unstructured textual information. It helps in transforming unstructured text into structured data with meaningful insights.
Text analytics involves not only extracting this unstructured text but also understanding it within its context.
To clarify further:
- Structured data refers to well-organized information.
- Text data is simply any form of written language.
- Unstructured texts are those without a predetermined format or structure.
Data mining analyzes structured information while dealing exclusively with unformatted texts falls under the domain of text mining. Text analytics goes beyond simply extracting unstructured information.
Text Mining and Text Analysis: A Comparative View
Although often used interchangeably, text mining and text analysis or text analytics serve separate functions within the field of data analysis. While text mining involves identifying patterns and extracting information from large amounts of textual data, text analytics software focuses on understanding and interpreting that extracted information in context.
In other words, while text mining provides a means to uncover “what” is present in the data through pattern recognition, it is up to the process of text analytics to determine “why” those patterns exist. Both text analysis and mining are crucial components when dealing with vast amounts of diverse language-based material such as electronic text documents or social media posts.
How Text Mining Diverges from Data Mining
Data mining or text data mining is primarily focused on obtaining insights from structured data, while text mining involves dealing with unstructured text data. This sets it apart as a distinct approach to other forms of analyzing information. The main challenge in text mining lies in converting this type of unorganized data into a structured format that enables effective analysis.
Despite the differences between text mining and data mining, the ultimate goal shared by both types of mining is extracting valuable insights from various datasets. Whether working with well-organized and manageable structural or complex unstructured textual information, these techniques are employed towards achieving meaningful conclusions for Use and understanding.
Text Mining in Action: Applications & Use Cases
Text mining plays a crucial role in improving business processes and enabling data-driven decision-making.
Since unstructured data comes from various sources, there is a diverse array of practical uses for text mining to derive information. Some of these are:
- Using text mining techniques to improve market knowledge
- Analyzing customer reviews to uncover sentiments, flaws, and improvement opportunities
- Text mining techniques are also used to extract information from text documents, automate data entry, and enable knowledge discovery
- Text analytics helps analyze clinical trial data, and identify patient sentiment towards treatments, and personalized care plans.
- Machine learning in text mining is crucial for extracting financial information from news articles, predicting market trends, and detecting patterns indicative of fraudulent activities like debit card fraud, payroll fraud and check fraud.
- Different text mining techniques are used by the government to analyze citizen feedback on policies, identify emerging issues, and improve public services
- Examining extensive amounts of unstructured data from different origins
- Text analysis also helps extract financial information from news articles, predict market trends, and detect fraud.
- Market intelligence using the machine learning capabilities of text mining software can be gained from online reviews. It can be used to comprehend client feedback better and evaluate their brand perception
Selecting the Right Text Mining Tools and Technologies
Choosing the right tools and technologies is crucial for leveraging the advantages of text mining. It can be challenging to determine which text mining software is best suited for your needs. Some important aspects to consider include user-friendliness, scalability, and compatibility with current systems.
Here is how you can choose the tool for mining text data:
- Define Your Requirements: Start by clearly defining your text mining objectives and requirements.
- Research Available Tools: Conduct thorough research to identify text mining tools that meet your requirements. Consider factors such as functionality, ease of use, scalability, performance, data compatibility, security, and cost.
- Evaluate Text Mining Algorithms & Models: Different tools have different text mining capabilities. Some widely used algorithms and models for text mining include decision trees, clustering algorithms, and neural networks.
- Check Compatibility and Integration: Determine whether the text mining tool is compatible with your existing systems, databases, and text data formats.
- Consider Customization and Flexibility: Choose a text mining tool that allows for customization and flexibility to adapt to your specific use case and requirements.
- Experiment & Evaluate: Test the functionality of the tools by taking free trials or demos.
Best Practices for Using Text Mining
It is evident that text mining has the capability to bring about significant changes in various aspects of a business. In order to fully capitalize on these benefits, businesses can utilize automation and streamline their operations, which will lead to time-saving, cost reduction and enhanced efficiency.
Through text mining businesses can gain a competitive advantage by identifying emerging trends, pinpointing potential opportunities and facilitating data-driven decision making.
1. Streamlining Operations with Automation
Integrating automation into text mining procedures can greatly increase the effectiveness of business operations by tackling monotonous and time-consuming tasks. Examples of such activities include sentiment analysis, topic modeling, keyword extraction, and summarization.
Automating these processes not only reduces workload but also enhances the precision of data analysis resulting in more reliable conclusions.
2. Gaining a Competitive Edge
Text mining has the potential to not only improve operational efficiency but also give businesses a strategic advantage. By examining their competitors’ strengths and weaknesses, companies can maintain a lead in the market. With text mining technology, businesses can evaluate their industry landscape, comprehend customer preferences, and identify emerging patterns.
As a result of these insights gained through text mining analysis, sound decisions can be made leading to improved business performance.
Get Started with Zonka Feedback
To sum up, text mining is a highly effective technique for converting unstructured text data into meaningful information. Its various applications span from enhancing customer satisfaction to revolutionizing the healthcare industry. It has become an indispensable asset in the field of data analysis and with proper selection of tools and utilization of automation, businesses can optimize their operations, gain a competitive edge, and make well-informed decisions based on valuable insights obtained through text mining. The potential uses for this technology are boundless.
Zonka Feedback offers powerful artificial intelligence, machine learning, and natural language understanding capabilities. It can be used as a complete solution for collecting customer feedback through surveys, polls, quizzes, and feedback forms. The feedback can then be analyzed to derive insights and make data-driven decisions.
Its text and sentiment analysis tools allow businesses to uncover hidden insights behind unstructured data received from text. Apart from text and sentiment analysis, the survey tool offers intent analysis, emotion detection, opinion mining, and other text mining algorithms to help businesses in extracting information and making the most of data.
You can sign up to get a free 14-day trial or schedule a demo to take a walkthrough of this product.
Frequently Asked Questions
1. What is text mining with example?
Unstructured text is widely present in business data, including customer feedback, online reviews, survey responses and emails. It entails the process of extracting valuable information from unorganized pieces of text, which could be identifying features in product descriptions or company names within a dataset. This type of data can provide important insights for businesses to analyze data and utilize for decision-making purposes.
2. Is it hard to learn text mining?
The concept of text mining, which falls under the umbrella of natural language processing, can seem intimidating initially. By breaking it down into fundamental principles and methods, it becomes easier to grasp and learn.
3. What is NLP and text mining?
Natural language processing, also known as NLP, is dedicated to comprehending the underlying meaning and organization of written text in order to improve translation accuracy across languages.
In contrast, text mining involves analyzing word connections, occurrences, and arrangements within a given body of writing. Both methods provide valuable insights into natural language but have distinct focuses and goals.
4. How is text mining typically done?
The process of text mining involves utilizing natural language processing (NLP) and artificial intelligence (AI) methods to automatically analyze inputted text, identify patterns, and organize it for evaluation and interpretation.
This presents both advantages and difficulties for companies dealing with vast amounts of data.
5. What is the difference between qualitative and quantitative data?
Qualitative and quantitative data differ in the sense that qualitative data depicts individual attributes and subjective reasoning, while quantitative data comprises numerical values that indicate the amount or quantity.