Imagine being able to peek inside the minds of your customers, effortlessly deciphering their hidden emotions, unspoken frustrations, and unbridled praise. Sounds like a marketer's fantasy, right?
That's what opinion mining brings to the table. It transforms unstructured text data into a treasure trove of insights, revealing the true opinions and points of view behind every feedback, like, comment, and review.
This blog gives a quick introduction to what opinion mining is, its major applications, and ways in which opinion is mined, the types of opinions you get, and what are the best sources for opinion mining. So, let's dive right into it.
TL;DR
- Opinion mining, leveraging NLP and ML, is crucial for interpreting sentiments in human language and offers businesses detailed insights into customer feedback and public sentiment by identifying key aspects of opinions such as their polarity (positive, negative, or neutral) and attributes.
- A variety of data sources are used for opinion mining, including customer feedback, reviews, social media posts, and survey responses.
- Opinion mining faces challenges such as dealing with multilingual data, sarcasm, and ambiguity, and requires sophisticated methods like context analysis and advanced algorithms for accurate sentiment and opinion classification.
- It is widely applied in business for brand monitoring, customer service enhancement, and market research.
- Zonka Feedback is one such opinion mining tool that you can use to get the outlook of people when it comes to your product, service, website, support staff, or brand as a whole. You can sign up with this advanced customer feedback tool for a 14-day free trial or schedule a demo to understand its opinion mining algorithm.
Measure Customer Feedback & User Insights
With Customer Feedback Surveys, understand the target audience's opinions and learn ways to delight your customers.
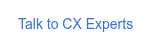
Understanding Opinion Mining
Opinion mining utilizes Natural Language Processing techniques and Machine Learning models to extract subjective information and analyze it from unstructured text data. It goes beyond simply identifying whether the text has positive or negative sentiment, but aims to capture the nuanced details of what people think, why they think it, and towards whom or what their opinions are directed.
It helps understand the structure and components of an opinion, like the target entity, the opinion holder, and the specific aspects being evaluated.
Now businesses can forget the days of agonizing over ambiguous feedback.
This isn't a guessing game – it's a data-driven adventure into the heart of customer sentiment.
With opinion mining as your guide, you'll crack the code behind their words, uncovering:
- The "why" behind the "what": Dive beyond surface-level opinions and understand the motivations, desires, and even frustrations driving them.
- Untapped opportunities: Identify previously hidden pain points and areas for improvement, catapulting your product or service ahead of the curve.
- A predictor of the future: Track sentiment shifts and emerging trends, predicting customer needs before they even know it.
- The secret sauce of success: Leverage these insights to craft targeted marketing campaigns, personalize customer interactions, and build lasting loyalty.
Elements of Opinion Mining That Help Analyze Customer Feedback
The primary elements of opinion mining include the identification of object features, opinion expressions, and opinion attributes, which are crucial for interpreting the sentiment of human language data.
Natural Language Processing: It is a vital cog in the opinion mining machine, enabling the classification of public opinions and the extraction of sentiments from the text, thereby effectively interpreting the emotional content of the language used.
Machine Learning: This bolsters opinion mining by facilitating the analysis of extensive textual data and spotting intricate patterns and sentiments. This automation of sentiment analysis and insight extraction from large-scale customer feedback is made possible through machine learning.
What's the Difference Between Opinion Mining and Sentiment Analysis?
While the terms opinion mining and sentiment analysis are often used interchangeably, they are not exactly the same. Sentiment analysis is the broader concept, generally referring to the process of determining the emotional tone behind a body of text. This is often a binary distinction, classifying the sentiment as positive or negative.
Opinion mining, however, digs deeper. It is a more nuanced form of sentiment analysis that goes beyond the general tone to understand opinions specifically or beliefs that lead to the overall sentiment. It involves dissecting the text to identify attributes or aspects being discussed and then assessing the sentiment related to each of these aspects.
For example, in a product review, sentiment analysis might give you an overall positive sentiment, but opinion mining will tell you that the customer loved the design and is inclined towards a cleaner user interface.
In essence, sentiment analysis gives you the "what" of the feeling, and opinion mining explains the "why" behind it, offering a more granular perspective on the various aspects that contribute to the overall sentiment.
The key distinction between opinion mining and sentiment analysis is done using semantic analysis.
Semantically, the manner in which people express their ideas is different from how they speak of their feelings. This distinction is crucial for opinion mining as it helps differentiate between factual statements and subjective emotions. By analyzing the semantics of language, we can gain a deeper understanding of the underlying opinions and sentiments.
For instance, the phrase "This phone has a 4K camera" is a factual statement, whereas "I adore the clarity of photos I take with this phone" expresses a positive sentiment associated with the product's feature.
Semantic analysis allows for this level of discernment, enhancing the accuracy of opinion mining.
Types of Opinions and Their Significance
Incorporating various types of opinions is significant in sentiment analysis as it enables a more nuanced comprehension of sentiment. Categorizing opinions into types such as positive, negative, or neutral allows businesses opinion extraction and get detailed insights into customer feedback, attitudes, and emotions, thereby empowering them to make informed decisions.
Here are the key types of opinions reflected in customer feedback:
- Positive opinions: These are expressions of satisfaction, approval, or happiness, are identified by their affirmative language and often include words like "love," "great," and "excellent." These sentiments can significantly boost a brand's image and are essential for understanding what a business is doing right.
- Negative opinions: These are marked by expressions of dissatisfaction, disapproval, or disappointment. They typically contain words such as "bad," "poor," or "terrible" and are critical for businesses to identify areas needing improvement or immediate attention. Opinion mining can also help identify emerging negative sentiments.
- Neutral opinions: These are those that neither express clear satisfaction nor dissatisfaction. They may reflect indifference or a middle-of-the-road stance and are often expressed with phrases like "average," "fair," or "adequate." These can be particularly valuable for identifying trends or shifts in customer sentiment over time.
- Mixed opinions: These opinions contain elements of both positive and negative sentiments and can be challenging to interpret. They may reflect a nuanced view or a conflicted experience, such as "I love the product's features, but the customer service was disappointing." Mixed opinions provide insight into the complex, multifaceted reactions customers can have to a product or service.
Data Sources for Opinion Mining
The data sources for opinion mining are largely based on customer feedback. Customer feedback is the raw material that opinion mining transforms into actionable insights. It's the voice of your customers, a treasure trove of opinions, thoughts, and experiences related to your product, service, or brand. But what are the data sources?
#1. Response from Surveys and Questionnaires
By harnessing the power of responses from surveys and feedback forms, businesses can gain valuable insights into customer sentiment, monitor concerns, gather extensive feedback, and decipher customer sentiments.
Surveys and feedback forms provide a robust platform for users to articulate their opinions and participate in dialogues on diverse topics. These platforms allow businesses to collect structured and quantitative data, enabling them to understand consumer sentiment on a deeper level.
Through the analysis of survey responses, organizations can gain a comprehensive understanding of customer preferences, pain points, and overall satisfaction.
Pros: Targeted data collection, specific insights on desired areas, quantifiable results.
Cons: Response rates can be low, potential for skewed results due to sampling bias, limited qualitative data.
Opinion mining insights: Customer satisfaction levels, feature priorities, specific pain points and improvements suggested.
#2. Online Reviews
Online reviews are a crucial component in opinion mining. They can be extracted from websites and analyzed for sentiment using natural language processing and machine learning algorithms such as Naïve Bayes classifier, Logistic Regression, and SentiWordNet.
The sentiments and opinions expressed in these reviews are vital for comprehending customer feedback, guiding product and service enhancements, and conducting competitive intelligence research.
Pros: Wide reach, diverse perspectives, easy accessibility, often detailed explanations.
Cons: Can be biased (positive or negative), potential for fake reviews, limited analysis depth.
Opinion mining insights: Sentiment analysis on product features, brand perception, comparative analysis with competitors.
#3. Social Media Posts, Mentions, and Comments
Social media platforms offer a plethora of data. These posts are another valuable resource for opinion mining as they facilitate real-time sentiment analysis and social media analysis across various social media platforms such as Facebook and Twitter, offering businesses the opportunity to access immediate and authentic consumer feedback on a significant scale.
Pros: Real-time sentiment tracking, brand perception beyond your platform, diverse range of opinions with social media analysis.
Cons: Requires advanced filtering for noise and irrelevant mentions, potential for negativity bias, sentiment might not reflect direct product experience.
Opinion mining insights: Brand perception trends, emerging issues or concerns, competitor comparisons, emotional response to marketing campaigns.
#4. Support Tickets and Live Chat Transcripts
Support tickets are a valuable source of data for opinion mining. These tickets provide in-depth insights into customer issues and frustrations, offering a direct feedback channel for evaluating the customer service experience.
By analyzing support tickets, businesses can identify common customer frustrations, assess the effectiveness of their support solutions, and pinpoint service bottlenecks.
Pros: In-depth customer issues and frustrations, direct feedback on customer service experience.
Cons: Focus on negative experiences, limited scope to overall sentiment, requires specific data extraction tools.
Opinion mining insights: Identify service bottlenecks, assess effectiveness of support solutions, understand common customer frustrations.
Analyzing these data sources enables organizations to:
- Comprehend consumer sentiment
- Monitor customer concerns
- Gather extensive feedback
- Decipher customer sentiments
This contributes to a comprehensive understanding of human language and public opinion.
What are the Different Opinion Mining Techniques?
Opinion mining involves the use of various techniques such as Natural Language Processing techniques, Aspect-Based Sentiment Analysis, and Machine Learning Algorithms.
These techniques help in recognizing the emotional tone and classifying opinions related to a product, service, or concept, categorizing data by aspect and determining the sentiment associated with each aspect, and employing algorithms to categorize sentiments and forecast emotional tones in text data, respectively.
Let us explore each of these techniques in further detail.
#1. Natural Language Processing
NLP is a significant part of opinion mining, using techniques to autonomously analyze and extract opinions from text data.
It involves processing text, interpreting language structure and context, and using machine learning for sentiment classification.
Techniques include sentiment analysis, named entity recognition, text summary, topic modeling, text classification, and keyword extraction. NLP contributes to sentiment analysis, providing valuable insights for businesses to monitor and understand sentiment associated with their brand and products.
#2. Aspect-Based Sentiment Analysis
Aspect-Based Sentiment Analysis (ABSA) holds a significant position in opinion mining.
Unlike general sentiment analysis, which primarily focuses on the overall sentiment expressed in the text, ABSA involves categorizing data based on specific aspects and determining the sentiment associated with each aspect.
This technique operates by categorizing data into specific aspects and subsequently identifying and evaluating the sentiment associated with each of those aspects.
#3. Machine Learning Algorithms
Machine learning algorithms, including both supervised and unsupervised techniques, are used to classify and predict sentiment in opinion mining. The various types of sentiment analysis and opinion mining algorithms utilized in opinion mining encompass Support Vector Machines (SVMs), deep learning algorithms, and algorithms that extract text features to identify sentiment, such as the bag-of-words approach.
- Supervised learning algorithms are employed in opinion mining to determine the sentiment of feedback by utilizing predetermined features. These algorithms are usually trained using a labeled dataset and can employ a variety of classification techniques to classify text as positive, negative, or neutral.
- Unsupervised Learning in Opinion Mining commonly entails the utilization of Topic Modeling Algorithms, which are unsupervised machine learning methods that have shown effectiveness in the field of Aspect-based Opinion Mining.
Furthermore, classification is utilized to categorize sentiments in text as positive, negative, or neutral, and prediction plays a crucial role in forecasting the sentiment or emotional content of text, providing valuable insights for businesses in analyzing public opinion, customer support, and automating tasks.
Applications of Opinion Mining in Business
Opinion mining has numerous applications in business. It contributes significantly to brand awareness by monitoring brand reputation, popularity, and consumer reception of new products or features. This understanding of opinions and feedback enables businesses to improve brand awareness and subsequently increase revenue.
In addition to brand awareness, opinion mining also plays a crucial role in the analysis of customer feedback by:
- Gathering and evaluating substantial amounts of unstructured data from diverse origin
- Enabling businesses to monitor current customer feedback and emotions
- Classifying text according to specific aspects such as usability, features, and shipping
- Leading to a more profound comprehension and actionable insights.
Market Research
- Understanding customer sentiment towards products and services: Analyze customer reviews, social media posts, and surveys to understand what customers like and dislike, identify areas for improvement, and gauge overall satisfaction.
- Examining competitor brands: Track competitor reviews and online discussions to understand their strengths and weaknesses, identify competitive gaps, and inform your own marketing strategies.
- Identifying emerging trends: Analyze public opinion on various topics to identify emerging trends and consumer preferences, helping businesses stay ahead of the curve.
Product Development
- Gathering feedback on new product ideas: Analyze reactions to prototypes and mockups to understand user preferences and refine product features before launch.
- Tracking user experience: Analyze app reviews and feedback to identify usability issues and improve the user experience.
- Prioritizing feature development: Analyze user feedback to understand which features are most desired and prioritize development accordingly.
Customer Service
- Identifying dissatisfied customers: Use opinion mining to proactively identify negative customer feedback on social media platforms and other channels, allowing you to address concerns promptly and improve customer satisfaction.
- Personalizing customer interactions: Analyze customer communication history to understand their sentiment and tailor interactions accordingly, leading to more positive customer experiences.
- Measuring the effectiveness of customer service initiatives: Analyze feedback after service interactions to gauge the impact of customer service efforts and identify areas for improvement.
Brand Reputation Management
- Tracking brand sentiment: Monitor online mentions of your brand to understand public opinion or perception and identify potential reputational risks.
- Identifying and addressing negative feedback: Identify negative comments and respond quickly and effectively to mitigate potential damage and protect your brand reputation.
- Measuring the effectiveness of marketing campaigns: Analyze sentiment before and after campaigns to understand opinions and their impact on brand perception and adjust strategies accordingly.
Overcoming Challenges in Opinion Mining
Despite its powerful capabilities, opinion mining comes with its own set of challenges. One of these is the handling of multilingual data. This can be addressed by employing data scientists to explore cultural differences in emoji usage in relation to sentiment and by conducting document-based emotion mining which relies on word representation and composition analysis.
Another challenge in opinion mining is dealing with sarcasm and ambiguity. This can be managed by utilizing techniques such as:
- Word sense disambiguation
- Entity recognition
- Coreference resolution
- Context analysis
Along with analyzing sentences individually and employing tools like Named Entity Recognition (NER) and Dependency Parsing. To ensure precise classification of opinions, a combination of rule-based analysis and machine learning-based techniques can be used to examine and differentiate opinions.
Getting Started with Opinion Mining
Opinion mining is a powerful tool for businesses to harness customer feedback and drive growth.
By utilizing Natural Language Processing, Aspect-Based Analysis, and Machine Learning Algorithms, businesses can extract valuable insights from text data and make well-informed decisions.
Challenges in handling multilingual data and dealing with sarcasm and ambiguity can be overcome with advanced techniques and expertise.
The power of opinion mining in driving business growth and improving customer satisfaction is best demonstrated through successful implementations of opinion mining tools like Zonka Feedback. As we continue to generate more and more text data, the importance and relevance of opinion mining will only continue to grow.
Zonka Feedback is a powerful tool that lets you analyze the emotions and opinions of customers presented in feedback through its AI algorithms. You can sign up for the customer feedback software for a 14-day free trial or schedule a demo to learn more about it.