What do your customers really think of you?
This has been a question that many businesses struggle with. In search of answers, organizations try many methods ranging from conducting surveys to monitoring online reviews. Whilst these are useful, there has never been a sure-fire way of knowing what customers truly think.
That is, until now.
Introducing customer sentiment analysis - a window into the innermost thoughts of the customer. But what exactly, is sentiment analysis, and more importantly, how it can boost customer experiences?
We’ll explore all that in this article.
TL;DR
- Customer sentiment analysis enables businesses to understand their customer's thoughts.
- Sentiment analysis, powered by technologies like NLP and machine learning, decodes customer emotions from various sources like customer feedback, reviews, and social media. It's not just about positive or negative; it delves into nuances.
- It transforms customer service, helps identify pain points, and allows personalized interactions.
- You can perform sentiment analysis through various approaches. From emotion analysis to multilingual analysis. It's not just about analyzing sentiments; it's about crafting a better customer journey and staying ahead of trends.
- Survey software becomes a potent tool, collecting and analyzing data, offering real-time insights, and paving the way for actionable improvements.
- Zonka Feedback is a powerful survey tool that comes with sentiment analytics to decipher hidden insights from the feedback received. You can sign up for a free 14-day trial or schedule a demo to learn more about this tool.
Measure Customer Feedback & Insights
With feedback surveys, understand what users need and learn ways to delight your customers.
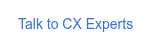
What is Sentiment Analysis?
From happy to sad, from disappointed to delighted, from agitated to satisfied, customers display an array of emotions and sentiments while interacting with a brand. And all of these emotions, combined, reflect upon your brand's customer experience.
In simple words, sentiment analysis is the technique used for identifying human emotions or sentiments behind the words they use. The process includes analyzing text data input by customers and finding out whether they are happy/positive, unhappy/negative, or neutral/passive.
Online, customers leave their thoughts in many different places. This could be through reviews, emails, and social media. Customer sentiment analytics software analyzes these messages to understand the emotions being expressed. Does a customer display positive, negative, or neutral emotions?
Sentiment analytics tools make use of a technique called natural language processing (NLP). This is a subset of machine learning designed to replicate the way humans interpret language.
With sentiment analysis, the text is assigned a score between -100 and 100.
The lowest numbers are negative, whilst the highest are positive. Anything that falls around the 0 mark is considered neutral.
In context to customer customer feedback. sentiment analysis helps analyze the context, emotions, and sentiments of customers based on the feedback that they share.
Importance of Sentiment Analysis in Improving Customer Experience
Sentiment analysis plays a vital role in enhancing the overall customer experience. By leveraging advanced technologies such as natural language processing and machine learning, businesses can gain valuable insights into the emotions and attitudes of their customers.
Here are some key reasons why sentiment analysis is crucial for improving customer experience:
1. Better Service Outcomes and Customer Experience
The best part about sentiment analysis is that it provides real-time insights into how customers perceive the brand, its services, and interactions.
Not only does it disclose the surface level of the feedback but also gives an understanding of the customer's sentiments and emotions while interacting.
By identifying positive or negative emotions through sentiment analysis, businesses can take proactive measures to address any issues or concerns, resulting in better service outcomes and an improved overall customer experience. They can adjust service strategies, product experience, and more based on how customers are reacting.
2. Complete Picture of Customer Sentiments
Sentiment analysis provides businesses with a comprehensive understanding of customer sentiments. It goes beyond just analyzing whether feedback is positive, negative, or neutral. It also aggregates sentiments from various sources, providing a comprehensive view.
No matter what the source of customer feedback, sentiment analysis tools will help you decipher the sentiments behind every word or comment.
By delving deeper into the emotions, attitudes, and intentions expressed in customer feedback, businesses can gain a complete picture of customer's negative and positive sentiments and tailor their strategies accordingly.
3. Understand the Customer’s Mood, Tone, and Attitude
Sentiment analysis allows businesses to discern the mood, tone, and attitude of customers. This understanding is crucial for providing personalized and empathetic support. By analyzing the emotions expressed in customer interactions, businesses can respond appropriately and effectively to meet customer needs and expectations.
This analysis also enables businesses to understand how the customers are feeling while they interact with your brand. It gives a better idea of the way you should respond to their feedback, enabling you to be more empathetic.
4. Enhance Customer Satisfaction based on Data-driven Actions
By analyzing customer sentiments, businesses gain valuable insights into the areas where they can improve and take data-driven actions to enhance customer satisfaction. Sentiment analysis serves as a guiding compass for businesses, allowing them to prioritize their efforts and allocate resources where they are most needed.
This approach of having customer satisfaction surveys to gauge feedback and sentiments leads to more efficient and effective customer service. It lets businesses focus on addressing the pain points that have the greatest impact on customer satisfaction.
5. Identify Customer Pain Points
Sentiment analysis helps businesses identify common pain points and issues faced by customers. By analyzing feedback and sentiments related to specific aspects, features, touchpoints, or stages in the journey of a product or service, businesses can pinpoint areas for improvement. This enables them to address customer concerns and enhance the overall customer experience.
By understanding the emotions behind customer feedback, you can pinpoint exactly where things might be going wrong. Whether it's a glitch in the system, a confusing process, or a feature that's more frustrating than helpful, sentiment analysis can highlight those pain points so you can smooth them out.
6. Offer Personalized Interaction
Sentiment analysis adds a personal touch to customer interactions by allowing businesses to tune in to the emotional vibes of their customers. It's like having a sixth sense that helps you respond not just to what customers say, but how they feel.
Sentiment analysis enables businesses to personalize their interactions with customers based on their sentiments. By tailoring responses and support according to the emotions expressed, businesses can create a more engaging and satisfying customer experience. It helps businesses speak the emotional language of their customers. It's not just about solving problems; it's about connecting on a human level.
Personalizing interactions based on sentiments builds a stronger bond between the customer and the brand, making the overall experience more memorable and satisfying.
7. Strategize Market Positioning through Informed Insights
Knowing where to position your product in the market is key to success. Making an informed decision, though, isn’t easy, and any misjudgment can sink your business. Luckily, constant monitoring of customer sentiment can give you the insights you need.
Not only will you get a better understanding of the features that customers would like to see in your products, but based on sentiment from different platforms, you can pick the best place to market your product.
8. Identify Churn Risks to Boost Retention
Sentiment analysis helps businesses identify customers who are at risk of churning. By analyzing the sentiments expressed in customer feedback, businesses can proactively address any issues or concerns and take measures to retain valuable customers.
Imagine a customer expressing frustration or disappointment consistently in their interactions with your brand. Sentiment analysis flags this as a potential churn risk. Instead of waiting for a customer to outright say, "I'm leaving," you can identify signs of dissatisfaction early on.
By monitoring sentiments, businesses can proactively address issues before they escalate. If a customer is unhappy, you can swoop in with personalized solutions, discounts, or even just a heartfelt apology.
This proactive approach not only resolves the immediate problem but also signals to the customer that their concerns are valued, potentially turning a disgruntled customer into a loyal one.
Use Cases of Sentiment Analysis in Improving Customer Experience
There are several instances when customer sentiment analysis helps businesses boost their overall customer experience. By leveraging sentiment analysis tools and techniques, businesses can gain valuable insights into customer emotions and perceptions, allowing them to make informed decisions and take appropriate actions to enhance the customer journey.
1. Proactive Issue Resolution
Sentiment analysis is a powerful tool that allows businesses to identify negative sentiments in customer feedback with ease. By analyzing customer sentiments, businesses can proactively address issues, resolve complaints, and mitigate potential escalations before they impact a larger portion of the customer base.
When any negative sentiment is detected, businesses can take immediate action to rectify the situation and provide a satisfactory resolution.
This proactive approach not only prevents further dissatisfaction among customers but also helps in building trust and loyalty.
Furthermore, by using sentiment analysis to identify negative sentiments, businesses can uncover patterns or common issues that may be affecting multiple customers. This allows them to implement systemic changes or improvements to their products or services, resulting in an enhanced customer experience overall.
2. Enhanced Customer Service
Real-time sentiment analysis is a game-changer for customer service teams. By identifying sentiments in customer interactions, businesses can provide personalized and empathetic support that truly resonates with their customers. When positive sentiments are detected, customer service representatives have the opportunity to acknowledge and reinforce them, further enhancing the customer experience.
With real-time sentiment analysis, customer service teams can tailor their responses based on the emotions expressed by customers. Whether a customer is frustrated, delighted, or somewhere in between, the team can adjust their tone and approach accordingly. This level of personalization allows businesses to build stronger relationships with their customers and foster a sense of trust and loyalty.
Furthermore, real-time sentiment analysis enables customer service teams to address potential issues before they escalate. By promptly identifying negative sentiments, teams can take immediate action to rectify the situation and provide a satisfactory resolution. This proactive approach not only prevents further dissatisfaction among customers but also helps in building trust and loyalty.
3. Brand Reputation Management
By leveraging sentiment analysis tools, businesses can effectively monitor online mentions and reviews related to their brand. This includes tracking social media posts, customer reviews on websites, and even comments on blog posts or articles. The sentiment analysis algorithms analyze the language and tone used in these mentions and reviews to determine whether they are positive, negative, or neutral.
Addressing negative sentiments promptly is crucial for reputation management. When customers express dissatisfaction or disappointment online, it can quickly spread and influence others' opinions of the brand. By responding strategically to these negative sentiments, businesses can not only address the concerns of the individual customer but also demonstrate their commitment to customer satisfaction to a wider audience.
Strategically responding to negative sentiments also provides an opportunity for businesses to turn a negative experience into a positive one. By acknowledging the customer's concerns and offering a solution or apology, businesses can show their dedication to resolving issues and building customer trust. This proactive approach not only helps in managing the immediate reputation risk but also contributes to long-term brand loyalty.
4. Continuous Product & Service Improvement
Organizations can identify areas for improvement, gather insights for product development, and prioritize enhancements based on customer sentiments.
Sentiment analysis provides organizations with a valuable tool for continuous improvement. By analyzing customer feedback, businesses can uncover common themes and trends that emerge from their sentiment analysis. These themes can be related to product features, customer service surveys and their interactions, or overall customer satisfaction.
Identifying these common themes allows organizations to pinpoint areas for improvement in their products or services. For example, if customers consistently express dissatisfaction with a specific feature, businesses can prioritize enhancements or updates to address these concerns. By listening to customer sentiments, organizations can gather insights that inform their product development roadmap and ensure that their offerings align with customer expectations and preferences.
5. Efficient Resource Allocation
Businesses can allocate resources efficiently by prioritizing actions based on the sentiments expressed. Focus on addressing critical issues and allocating resources where they are most needed.
Efficient resource allocation is crucial for effective business management. By using sentiment analysis to categorize feedback based on urgency and importance, businesses can optimize their resource allocation. This allows them to prioritize actions based on customer sentiments, addressing critical issues promptly and allocating resources where needed.
Sentiment analysis also helps businesses allocate resources strategically for enhancing customer experience, such as investing in training for customer service representatives or improving product features.
6. Customer Journey Optimization
Organizations can optimize the customer journey by identifying pain points and positive experiences. This leads to a more seamless and satisfying customer experience.
At each touchpoint, sentiment analysis can be applied to analyze customer feedback and interactions, providing organizations with a comprehensive understanding of the customer's sentiment throughout their journey.
For example, let's consider a scenario where a customer is browsing an e-commerce website and encounters difficulties in finding the desired product.
By using voice of customer tools that analyze customer sentiments, organizations can identify pain points and frustrations, allowing them to improve the website's user interface, search functionality, and product categorization for a better browsing experience.
Similarly, sentiment analysis can be applied to other touchpoints in the customer journey, such as the checkout process, customer support interactions, or post-purchase follow-ups. By continuously monitoring sentiments at these touchpoints, organizations can proactively address any issues or concerns, providing timely and personalized solutions to enhance the customer experience.
7. Forecasting Customer Trends
By identifying evolving customer preferences, businesses can forecast trends and align their strategies with changing market dynamics.
By analyzing the sentiments expressed by customers in their feedback, businesses can gain valuable insights into evolving customer preferences. Sentiment analysis algorithms can identify patterns and trends in the language, tone, and context of customer feedback, providing businesses with a comprehensive understanding of the shifting market dynamics.
For example, sentiment analysis may reveal a growing sentiment towards eco-friendly products and sustainability.
By detecting this emerging trend, businesses can proactively adjust their product offerings and marketing strategies to cater to the increasing demand for environmentally conscious options. This could involve developing new sustainable product lines, incorporating eco-friendly materials, or promoting green initiatives.
Furthermore, sentiment analysis can also uncover emerging trends in customer expectations and experiences. For instance, if a significant number of customers express dissatisfaction with the speed of customer service responses, businesses can anticipate a growing demand for faster and more efficient support. Armed with this knowledge, businesses can invest in improving their customer service infrastructure, such as implementing chatbots or expanding their support team, to meet the evolving expectations of their customers.
8. Benchmark offerings in a competitive landscape
Businesses gain insights into how their offerings are perceived relative to competitors. This benchmarking helps in adjusting strategies to stay competitive and improve market positioning.
Sentiment analytics tools not only allow you to monitor the sentiment of your customers but also your competitors’ customers. What do they like about the competitor's product? What would they like to see improved? Knowing the answers to these questions is key to gaining a competitive advantage.
This, in fact, can uncover valuable insights to help drive the direction of future product development. With any luck, in the process, you’ll also pull customers away from competitors and towards your business.
For example, if sentiment analysis reveals that customers consistently praise a competitor's fast delivery times, a business can prioritize improving its own delivery speed to stay competitive.
Alternatively, if sentiment analysis shows that customers value the personalized customer service provided by a competitor, a business can invest in training their customer service team to offer a similar level of personalized support.
By benchmarking offerings against competitors through sentiment analysis, businesses can strategically position themselves in the market and differentiate their products or services based on customer preferences and expectations. This allows them to stay ahead of the competition and attract and retain customers by offering unique value propositions.
Types of Sentiment Analysis
Data collected from customer sentiment analytics tools is crucial for all types of decision-making. However, to make the most of this data, businesses also need to understand the different types of data that they can collect with such tools.
Some of these are discussed below:
- Binary Sentiment Analysis: Binary sentiment analysis classifies text into two categories: positive or negative. This sentiment analytics approach is commonly used for straightforward sentiment identification, such as determining whether a customer review is positive or negative.
- Multiclass Sentiment Analysis: Multiclass sentiment analysis involves classifying text into more than two categories, often including positive, negative, and neutral, as well as additional sentiments. This is useful for scenarios where a nuanced understanding of sentiments is required, such as categorizing feedback collected from a 1 to 5 rating survey or a 1 to 7 rating scale survey into strongly positive, mildly positive, neutral, mildly negative, and strongly negative.
- Aspect-based Sentiment Analysis: Aspect-based sentiment analysis goes beyond overall sentiment and analyzes sentiments related to specific aspects or features mentioned in the text. It is ideal for understanding sentiments associated with different features of a product or service, helping businesses pinpoint areas for improvement.
- Emotion Detection: Emotion detection identifies specific emotions expressed in text, such as joy, anger, sadness, or surprise. It is valuable for understanding the emotional tone of feedback, enabling businesses to respond appropriately and cater to emotional nuances.
- Intent Analysis with Sentiment Analytics Tools: Intent analysis focuses on identifying the intentions or goals expressed in the text. Useful for understanding what customers are trying to achieve through their communication, helping businesses tailor responses and services accordingly.
- Graded Sentiment Analysis: Graded sentiment analysis assigns a numerical score or grade to sentiments, providing a more fine-grained understanding of intensity. This type of sentiment analysis enables businesses to quantify the strength of sentiments, allowing for a more nuanced interpretation of feedback.
- Multilingual Sentiment Analysis: Multilingual sentiment analysis involves analyzing sentiments in text written in multiple languages. It is essential for businesses operating globally, as it allows for the analysis of sentiments across diverse linguistic landscapes.
Ways to Analyze Customer Sentiments & Technologies Behind It
While the sentiment analysis model offers a clear picture of what the customers are feeling toward your business, product, or service, there are different ways in which you can analyze it to create the models. Whether you are assessing positive sentiment or negative sentiment, here are the technologies that are powering every customer sentiment analysis tool.
Technologies Powering Customer Sentiment Analytics Software
The field of customer sentiment analysis is constantly evolving, and businesses have access to a range of technologies that power these analysis tools. These technologies leverage advanced algorithms and techniques to accurately analyze and interpret customer sentiments.
- Natural Language Processing: NLP is like the brain of the operation. It helps computers understand, interpret, and generate human language. When it comes to sentiment analysis, NLP is crucial for parsing through vast amounts of textual data—like customer reviews, social media comments, and support tickets—to extract meaning and identify sentiments.
- Natural Language Understanding: This is like NLP's sophisticated sibling. While NLP focuses on language processing, NLU goes a step further, aiming to comprehend the meaning behind the words. For sentiment analysis, NLU helps in understanding the nuances of human language, recognizing sarcasm, context, and the subtleties that can completely change the sentiment of a statement.
- Machine Learning Artificial Intelligence: ML and AI are the powerhouses that make sentiment analysis smart. Machine learning algorithms can be trained on vast datasets to recognize patterns and nuances in language that indicate different sentiments. AI, often in the form of neural networks, enhances the learning capabilities, enabling the system to adapt and improve over time.
Approaches To Mine Customer Sentiments
When it comes to mining customer sentiments, there are several approaches in addition to the technologies that you can use. These could be aspect-based sentiment analysis, opinion mining, rule-based systems to analyze sentiment, and many more.
Here are the most popular approaches to analyze sentiments from positive and negative words received from your customers in the form of unstructured data.
1. Opinion Mining
Opinion mining involves using natural language processing and machine learning techniques to identify and extract subjective information from textual data. It also analyzes features like word frequency, sentiment lexicons, and context for training models to recognize sentiment patterns. This type of sentiment analysis can also identify the entities in the text, such as product names or brands, to enhance the accuracy of sentiment analysis.
2. Rule-based Sentiment Analysis
Rule-based sentiment analysis involves the use of predefined rules and patterns to determine sentiment in text. These rules can be crafted manually or derived from linguistic analysis into sentiment analysis tools. It requires creating rules that consider grammatical structures, negations, and intensifiers to infer sentiment.
There are several advantages of using the rule-based sentiment analysis approach. It are often more interpretable, allowing analysts to understand how sentiment decisions are made. Further, the rules can be tailored to specific industries or domains, making them more accurate in certain contexts.
3. Customer Emotion Analysis
Emotion analysis goes beyond a simple positive or negative sentiment classification. It delves into the finer nuances of emotions—joy, frustration, anger, satisfaction, etc. This approach helps businesses not only identify if customers are happy or unhappy but also understand the specific emotions driving those sentiments.
4. Multilingual Sentiment Analysis
In our interconnected world, businesses often cater to customers from diverse linguistic backgrounds. Multilingual sentiment analysis ensures that customer sentiments are accurately understood and analyzed regardless of the language used. This approach is crucial for companies with an international presence or those targeting a multilingual customer base.
5. Feedback Loop for Continuous Learning
A feedback loop is like the heartbeat of sentiment analysis systems. It involves continuously feeding new data and insights back into the system. This iterative process allows the sentiment analysis system to adapt and improve over time. As customer sentiments evolve, the system learns to recognize new patterns, words, or expressions, ensuring that it stays relevant and accurate.
Performing Customer Sentiment Analysis with Survey Software
When you decide to perform sentiment analysis on the customer feedback received, one of the most effective ways to do the same is by using survey software.
These sentiment analysis tools in survey software are powered with NLPs, deep learning, AI/ML technology, and much more. They can perform accurate sentiment analysis and get an in-depth insight into the underlying sentiment behind every feedback.
1. Create Surveys with open-ended Question
Open-ended questions allow customers to express themselves freely. This qualitative data is gold for sentiment analysis tools. It provides rich insights into the nuances of customer sentiments rather than restricting them to predefined choices.
2. Distribute Surveys Across Multiple Channels
Distributing surveys through various channels ensures that you capture feedback from a diverse range of customers. Whether it's email, social media, or your website, a multi-channel approach helps you gather a representative sample of customer sentiments.
3. Collect Customer Feedback or Data
Modern survey software enables businesses to collect customer feedback in real time, providing them with valuable insights into the evolving sentiments of their customers. This immediacy is crucial for businesses that strive to stay agile and responsive in today's fast-paced market.
By leveraging modern survey software, businesses can gain a pulse on customer sentiments as they change over time.
The customer feedback tool allows for the collection of feedback from multiple channels, such as email, social media, and websites, ensuring that a diverse range of customers' opinions are captured. This comprehensive approach provides a holistic view of customer sentiments, allowing businesses to make informed decisions and take immediate action.
Real-time data collection empowers businesses to stay ahead of the curve by quickly identifying emerging trends and customer preferences. With this information at their fingertips, businesses can adapt their strategies and offerings accordingly, ensuring that they remain competitive and meet the evolving needs of their customers.
4. Automated Analysis
Survey software often comes with embedded analytics capabilities, making it a powerful tool for businesses to gain valuable insights from customer feedback software. One of the key features of survey software is its automated sentiment analysis tools, which can sift through large volumes of responses quickly and categorize them based on sentiments.
Automated sentiment analysis is invaluable for businesses dealing with high volumes of customer feedback. It saves time and resources by automating the process, allowing businesses to process large amounts of data efficiently. Using advanced natural language processing and machine learning algorithms, these tools accurately determine sentiments expressed in each response, including nuanced emotions like joy, frustration, anger, or satisfaction.
Categorizing responses based on sentiments helps identify trends and patterns in customer feedback, enabling businesses to prioritize improvements and capitalize on strengths.
This data-driven approach enhances products, services, and customer experience.
5. Visual Reports
Visual feedback tools help in transforming raw data into visual reports making it easier to understand and communicate trends. Visual representations, such as charts and graphs, offer a quick and intuitive way to grasp the overall sentiment landscape.
- Word Count: Word count analysis helps identify frequently mentioned words or phrases, highlighting key themes in customer feedback. This quantitative approach provides a structured way to understand the most prevalent sentiments.
- Word Cloud: Word clouds visually represent the frequency of words, with more prominent words indicating higher usage. This offers a quick glance at the most common sentiments and issues expressed by customers.
- Tags (Positive, Negative, Neutral): Automated tagging of responses into positive, negative, or neutral categories simplifies the analysis process. It provides a high-level overview of the overall sentiment distribution.
- Cumulative NPS (for repeated words): By analyzing repeated words and sentiments over time, you can track changes in NPS. This longitudinal analysis helps businesses understand if sentiment trends are improving or declining.
6. Take Actions
The ultimate goal of sentiment analysis is action. By analyzing customer sentiments and gaining valuable insights from survey responses, businesses can make informed decisions and take proactive steps to enhance the customer experience.
These insights provide a roadmap for implementing changes and improvements that align with customer preferences and expectations.
Whether it's addressing areas of dissatisfaction, identifying opportunities for innovation, or refining existing processes, sentiment analysis serves as a catalyst for positive change. It empowers businesses to prioritize customer needs, tailor their products or services accordingly, and ultimately foster stronger customer relationships.
By leveraging the power of sentiment analysis, businesses can take actions that not only address immediate concerns but also drive long-term growth and success.
7. Close Feedback Loop
Closing the feedback loop involves responding to customers based on their feedback. Acknowledge their input, communicate changes you've made, and show customers that their opinions matter.
Zonka Feedback for Customer Sentiment Analysis
Zonka Feedback is a powerful survey software that can help you build a sentiment analytics system that help decipher real customer emotions from the feedback received. It helps collect customer feedback at different stages of the customer journey through various survey channels.
Once you have the feedback, its sentiment analytics system automatically deciphers the intent behind the feedback and gives an in-depth report.
You can sign up with this online survey tool to start collecting feedback with its 14-day free trial or you can simply schedule a demo to understand how Zonka Feedback can help with sentiment analysis.